NeurIPS 2024 - Posters and Hallways 3
So, hey, I'm Kelvie Zomorak. I'm I work at INHERA, an EFP Energy Nobel in Paris, France. And this is the joint work of Anna Boujee, I mean, the the last time we were introduced a new multi agent wind farm learning benchmark for wind farm control. So in wind farms, you have a phenomenon called WECT FX, where wind turbines operating upstream created by the wind condition for the turbines operating downstream. And we propose to cap this as a decentralized, partially observable market decision process, where, aim agents are controlling aim turbines to maximize a shared reward, which can be the total production, a distance of production target, or including loads and turbine structure.
Speaker 1:So our environment has 20 approaches from layouts inspired from real wind farms, taking into account three win condition scenarios, and, is plugged in with two different simulators, static and dynamic, which can allow us to evaluate transfer learning strategies from a static case to to dynamic case. I'm sure that's, switching from static learning to the going in dynamic condition is not that easy. And maybe try, robot shaping that we hope to get rid of with this benchmark.
Speaker 2:What is static and dynamic, refer to in this setting?
Speaker 1:Yeah. So in the static setting, we basically assume that the wind fill is constant in time. So when you evaluate the dynamics, you have to, assume that the dynamics is a succession of static states. Right? Whereas in the dynamic case, it's taken to account the evolution of the wind field, with with the time.
Speaker 1:And so yes. What we show is that when you assume that you're working inside the conditions, you actually lose a bit of the partially observable, property of your problem. Because when you look at the observation of every agent, we would be able to reconstruct the entire wind field, of of the wind form, which might explain why it's so hard to switch from static to dynamic keys.
Speaker 3:Hi. My name is Andrew Wagonmaker. I'm a post doc at UC Berkeley, and our paper is called overcoming the sim to real gap, leveraging simulation to learn to explore for real world RL. So the basic idea behind our paper is we wanna understand when naive sim to real transfer fails, if there's some better way we can use a simulator just to allow for efficient real world learning. And what we show theoretically, is that indeed there is.
Speaker 3:So, we we show that instead of using your simulator to learn to solve a task, the the goal task, and then transferring that to the real world, which is a standard policy standard practice or sim to real transfer, you should learn your use your simulators to learn a set of exploratory policies, transfer these exploratory policies to the real world, use these exploratory policies to explore in real world, and then use the data they collect to learn to solve your task in real. What we show theoretically is that the conditions on that succeeding are significantly weaker than the conditions on this direct sim to real transfer succeeding. In fact, we get an exponential gap in the complexity between these two methods. We also show in practice, we want run some actual sim to real experiments on a Frank or robot, and we find that this approach can yield, like, pretty significant gains in practice as well. Basically, transfer making tasks that were previously, infeasible into a feasible task.
Speaker 4:I'm Harley Wiltser. I'm a PhD student at, Mila and McGill University in this is some joint work that I did with Jesse Fairbrother, Arthur Gretton, and Mark Roland from MELA and Google DeepMind. And it's called foundations of multivariate distributional reinforcement learning. And the idea behind this paper was to come up with, tractable algorithms, that we could learn to make some model that we could use for distributional zero shot transfer in RL. So, for example, if you don't know while you're training what reward you wanna optimize, and you also don't know when you're training, what risk measure you wanna optimize.
Speaker 4:So, like, maybe you don't know how, risk sensitive you're gonna be while you're learning from the environment. Our our method introduces algorithms for, convergently learning, distributional successor features, which we show that you could then use downstream, to predict return return distributions over, a pretty broad class of reward functions. And you could use this, like, sort of, downstream for ranking policies based on arbitrary, like, risk preferences and reward functions.
Speaker 5:This is contextual bilevel reinforcement learning for incentive alignment, with authors Vincent Stoma. That's me. Varna Pastor, Andreas Krause, George Ramponi, and Iffan Hulm. We are considering a sort of Stackelberg RL problem where there's a leader that can modify the rewards and transitions of a mark of decision process. And we have a follower that, solves that Markov decision process with entropy regularization.
Speaker 5:What we are interested in is that the leader wants to minimize some loss function, some objective that's not necessarily aligned with the lower level. And what we can show is that we can actually compute the gradient exactly, of how the leader can minimize that loss, using, in particular, the, the the sort of entropy regularization that gives us a nice form of that gradient. The nice part of that is that, we can show that we can actually very cheaply get stochastic estimates of that gradient, and we can sort of very cheaply run stochastic gradient descent with the necessary convergence guarantees. On the more sort of application side or sort of the interesting, applications, include many economic problems, like tax design, principal agent problems, mechanism design, but also RLHF, where sort of the the upper level is a reward model that's sort of learning the optimal rewards, and the lower level is, for example, the language model that's learning the optimal policy with respect, to that reward.
Speaker 2:Can you can you talk about, what are the leader and follower in the different application areas besides RLHF, just to to help listeners understand?
Speaker 5:Of course. So in in contract design, the leader would be the the sort of principle that, cannot really take actions, but has some some interest some some objectives. And the, follow would be the agent that can act, and has its own interests and costs, but, is incentivized by the by the principle to do different things. In in mechanism design, there's actually a previous paper, from, from our group where, we show that you can, frame this as the same problem where the the leader is sort of the mechanism designer that sets certain parameters, and the follower is sort of a a a welfare maximizing agent that tries to, maximize and a fine transformation of social welfare given those parameters from the upper level.
Speaker 2:These two levels remind me a little bit about, of, feudal reinforcement learning, a concept that came up a few years ago. Is that is that something that that is related here?
Speaker 5:I I would need to check this out. Yeah.
Speaker 6:My name is Tony. I am from Columbia University. And in our paper, QGIM, we provide a scalable framework for simulating QN networks and benchmark QN networks. So QN has been traditionally a, a a operational research area, and people have been developing traditional heuristic policies for human systems. But recently, with rise of more complex human systems like, hospitals, orchestration, we've been looking at language model serving.
Speaker 6:When the service system get more complicated, people have been looking at using deep learning and reinforcement learning to, to train policies for queuing system. So what we've been we've been doing is providing a good simulator and a good platform for defining your network and plugging your policies to bridge this up to communities, to traditional Aura communities, and people who know about RL.
Speaker 7:Hi. I'm Leon. I'm also from Columbia University, and I'll be talking about the, RL baselines. So traditionally, the queuing policies are pretty much static and does not learn from data. And, because the complex top network topology and the system design in the real world, it's very, convenient and appealing for us to, study how those data driven policies, like reinforcement learning policies per, performs in the real world.
Speaker 7:So, what we find is that if you simply use a state of the art ROL policy, like PPO to, just plugging into any kind of, like, current problem, it'll perform poorly because of a lot of issue. And, we basically designed a specific action parameterization technique, tailored for, RL. And we find that, after with the action parameterization, we develop a new state of the art RO policy, called the PPOWC. We still find that even with that kinda, like, action parameterization where you scale up, in the network size, we still find that our policy performs sub optimally comparing to traditional policy. Means meaning that there's still a long way to go for us to develop truly scalable data driven decision making algorithm for queuing problems.
Speaker 2:So I got a simple question. What what is the observation space and action space, for for these environments?
Speaker 7:So for the observation space, it's basically just a queue lens of each of the queue. And the action space, you can define your own action space. But what we did is that we basically created a routing matrix. So if you have end server and end queue, it's gonna be, n times end matrix specifying, which server, is assigned to serve which queue. And, you can make some relaxation, make it, like, a continuous, probabilistic matrix, meaning that each row, is a probability simplex.
Speaker 7:But in the real world, it has to be discrete because you cannot say, like, a queue serves, queue a server serves q one for zero point zero of the time and 0.7 of the time.
Creators and Guests
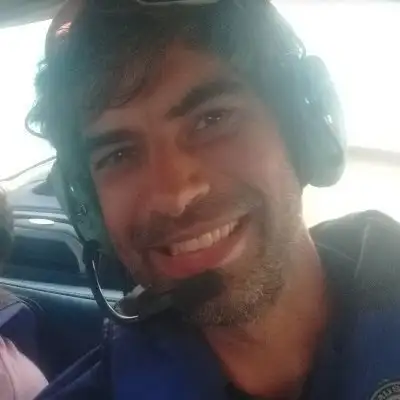
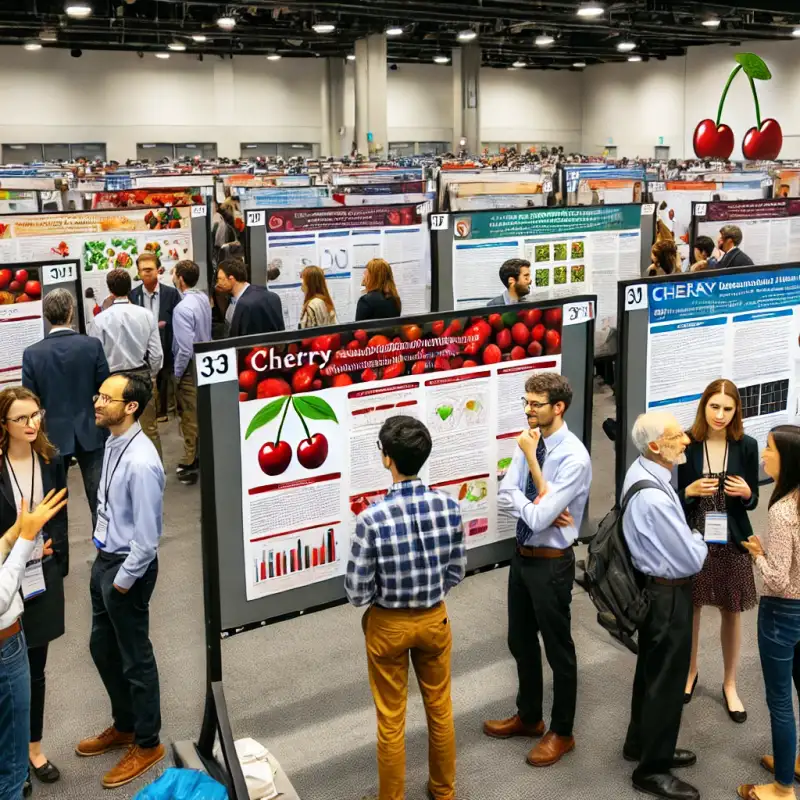