Glen Berseth on RL Conference
TalkRL Podcast is all reinforcement learning all the time, featuring brilliant guests, both research and applied. Join the conversation on Twitter at talkRL podcast. I'm your host, Robin Chauhan.
Robin:Today, I'm very pleased to have our guest, Glen Berseth. Glen is a assistant professor at the University of Montreal, a core academic member of the Mila Quebec AI Institute, Canada CIFAR AI chair, member of LINSTITUTE Courteos, and co director of the robotics in embodied AI Lab Less Real. Welcome, Glen. Thanks for being here.
Glen:Yeah. Thank you, Robin. Happy to be here.
Robin:So we're here to talk about, the new conference, the RL conference. Now it's not very often. It's a very rare event that a new conference is born, let alone an RL Conference. So I'm very excited about this. Can you tell us, what is the RL Conference and why did you create the RL Conference?
Glen:Yeah. The so the RL Conference is you know, the aim of this is really to get to and have a nice space for people that are like most passionate about the problems in reinforcement learning and like a priority space for a conference focused on those problems. And there's sort of a little bit of story around how this ended up being created. So I was at ICML last year and learned a little bit at the Deep Reinforcement Learning Workshop that's normally at NEURIPS, which is a fantastic place for a bunch of RL people to collect together and chat. Happened to not be planned.
Glen:Happened to also run into Amy and Eugene at the conference, and Amy had the idea of, why don't we just make an RL conference? And so I sort of, at least a little bit, you know, blame her for how this ended up happening, but I guess it was definitely her idea, and I spent think we all spent a few days thinking about it more and more and whether or not it made sense and really started to get into the idea. And so a lot of the reason we created it is because we know a lot that RL has a very specific set of problems that isn't always the primary focus of some of the larger conferences. So we really are trying to make that space, which has been, like, extremely helpful for a lot of other areas of research as well. Like there are many computer vision conferences that have really spurred a lot of development and focus on computer vision problems.
Glen:Could even be a reason that a lot of, you know, the pace of research and progress in computer vision was accelerated by having those conferences, being able to congregate, being able to mix ideas with the people that have really focused on the same problems.
Robin:Can you say more about RLC kind of in context of other related conferences like including RLDM? I guess you mentioned NeurIPS, the workshop specifically. But, yeah, RLDM and I guess the other, main ML conferences. Like, do they have a different type of focus on RL or a different type of scope for RL? Or is it were you kind of collecting, that same community that shows up at the other conferences, with more focus?
Glen:Yeah. So even first, I'll start out with, like even all the organizers for the RLC, we really, really love the RLDM conference. We don't want to see that go anywhere at all. It's a fantastic workshop, has a great space in the RLL community. The getting down to a few of the details is at least a bit somewhat traditionally, RLDM has been a great mix of having reinforcement learning and then a lot of like cognitive and bio inspired backgrounds and putting those 2 together for reinforcement learning.
Glen:So it has that type of theme for some of the conference. So in some respects, yeah, and related to at least RLDM, RLC wanted to be a bit more broad in terms of stuff for RL. So it's not as focused on having the background and mixing with a lot of people in the cognitive science areas as well, like the psychology areas. Not that those aren't fantastic areas to work, It's just we think RL is, like, even more broad and there's other areas that can focus throughout RLC. And it does make me think to go back a bit to that first comment, like related to like NeurIPS and some of the Deep RL workshop, what was also happening a bit there was really reinforcement learning has grown quite a bit.
Glen:And like this year when I went to NEURIPS there must have been 8 to 9 different workshops that were themed around reinforcement learning. So now there's even starting to become like some sub areas of reinforcement learning that can make their own workshops. So this community is growing quite a bit. So at the same time we want to have a space here for RLC for people to also be able to publish work. So like these workshops are great, but we also want to have a place where there's an area where we can submit things, we can publish stuff, students can get more credit for things when they're working on different areas.
Glen:So there was a lot of some of those choices that have gone into the way we've been working on designing this. And I gotta say, like, we've been I've been working on designing this with a bunch of fantastic people with Amy and Eugene and Scott, and Philip and Bruno. And it's like the passion and, like, ability, I think, for some of them to kinda, like, vent their thoughts about the research community and how to improve it through the RLC conference has been a really eye opening and fantastic experience to have.
Robin:So I see on the web page that, a lot of things are in scope. I was gonna ask about certain gray areas and I think they're all in scope, imitation learning, supervised alternatives to RL planning, other types of decision making. How do you generally describe kind of what is on the edge of of being in scope for the for this conference?
Glen:Yeah. Amongst the organizers, we've definitely had a lot of discussions about this, of what we consider reinforcement learning. I mean actually when we were getting to the beginning of this, you know, a kind of another possible name for the conference would be like the sequential decision making conference. And I'd usually describe things that would fit under that problem because that's the problem that we're actually trying to solve here. Like RL is a tool for being able to handle decision making problems.
Glen:So if there is like some portion of this that is some type of decision making that should fit inside of the umbrella of things, it'll work well for RLC and that people all have a strong interest for things related to sequential decision making. So imitation learning is still in many cases, figuring out how to make a bunch of decisions that will be able to imitate some other agent's behavior. And these days, because they're like, getting into some mix or at least there is a gray barrier around some supervised learning methods in RL these days, yeah, we're still open to those types of papers as well. Because even me personally, I'm very interested to understand, you know, what is the boundary between supervised learning methods, like sequence prediction methods that look a lot like reinforcement learning and can be applied to similar problems for RL or sequential decision making. And planning is still very much in the space of a sequential decision making, as well as, yeah, just general types of decision making.
Glen:So we've been trying to use that lens to include things for the conference. We haven't and we really don't plan to, like, apply things too judiciously. Like, if something can be seen as useful for RL people, like even better representations for making decision making, I would think those would be like featured and helpful at the conference. I don't want to necessarily say there's something that's totally outside the scope, but I prefer to talk about the things that are, like, more obviously inside the scope. And these are definitely some of the topic areas that we'd all be interested in.
Robin:So if you're on Twitter at all, we hear people complaining about the review process. What do you think of that problem? And do you guys have any, thoughts in that direction or or any changes for this conference?
Glen:Yes. We have a lot of thoughts in this direction. And actually, in some ways we've had to I wouldn't say pull back from some of our thoughts, but there were certainly some really extreme or out there ideas that would have been really fun to try. But another when we were putting together this conference, one of the things we were looking at is, can we make a better review process? There's already at least tens of other conferences out there.
Glen:We don't really want to add to the reviewer burden, which is already pretty high for a lot of people doing research in our area. So we took this really under consideration and tried to design like a reviewer process that will be like as much signal that we can get from the noise ratio, while reducing the amount of reviewer burden. Because we know at many conferences, you know, we tend to like submit papers, we get 3 to 4 reviews, and one paradigm that might be used at some other conferences is to be able to, okay, there's some noise that might be coming from the reviewer process. Well, maybe we should increase the number of reviewers. That'll help reduce variance.
Glen:This isn't always true in many cases. It's because as much as everyone in the group for RL, we're all kind of experts in sequential decision making, there's still a lot of like human aspects to some of these decisions that need to take into consideration. So if we burden this fantastic group of researchers with more additional reviews, it's possible the quality of reviews go down and there's some arguments to say that is happening. So, we wanted to focus on a slightly different review process And I really have to think, like Martha White and Adam White, that have put together a lot of this already. So I'm going to kind of explain what they've put together to the best of my ability.
Glen:But we've done some discussions back and forth, but we've really found like a great middle ground to work with. But here is also it's the idea that we're actually going to have, for each paper, 2 reviewers. And the mental model for this is really when I go to review a paper myself, I like to think, Okay, no, I want to treat this like this is one of my other colleagues here at Mila right now. And my really goal is to provide them feedback that'll help them improve and get this paper accepted and do fantastic research. So with that in mind, what we've been looking into, what we've decided on is there's going to be 2 reviewers for each paper.
Glen:It's going to be what's called a senior reviewer. This is someone who's like really skilled in reinforcement learning, will know the topic area very well. We'll give the authors for the paper like really credible and strong feedback on all parts of the paper. And then there'll be one additional reviewer that's added to it that can be a little bit more general, maybe not an expert in that area. And the idea here is one thing that we kinda wanna avoid for this specific conference and what also adds adds a lot of noise to the review process, is the idea of trying to really judge, like, future value of a paper, which is a very tenuous and nebulous concept.
Glen:There are many papers at conferences that were barely snuck into conferences. Now they are some of like, the most highly cited papers. And it's especially difficult for, like, younger reviewers, even expert reviewers, to judge whether or not, you know, like a submitted paper is gonna truly be valuable into the future. So this isn't really a great measure to have and to base a lot on in terms of being able to accept a particular paper. The idea for the senior reviewer is they're the ones that will be there to provide the most detailed feedback, ensure that the paper is novel, it is technically correct, and that it is good.
Glen:And then one additional reviewer to make sure that we can get some more feedback, as well. That'll be more across papers. So this particular design and only having 2 reviewers is part of our design plans to basically get as much signal as we can from the reviewers, giving them less work to actually do and to provide even better feedback to the people that submit papers because that used to be a lot of the point When people submit papers to journals, a lot of the times it might work, but it was also to get comments and feedback from the community. That's good. This is supposed to be part of the process of supporting each other because we all wanna figure out the science together.
Robin:Sounds like you really thought about, how to make this decision under uncertainty and, how to limit the cost of your ensemble and also how to design your reward function. So makes a lot of sense.
Glen:Yeah. We're all decision making professionals. So sometimes maybe we've even spent too much time on this, but that's a fun practice to get into.
Robin:So do you have a a future vision for RLC, or is it just, let's get through this conference and let's see how it goes? Or is RLC gonna take over the world soon?
Glen:Well, so this also depends on who you ask from the kinda committee for the conference right now. But so some of the general things is there's definitely a lot of future plans. Actually when we announced the conference, like almost 5 seconds later, a lot of people from the European workshop on reinforcement learning got in touch with us. So there's at least some plans in the works to be able to have RLC in Europe next year. So we're working on co organizing with some of the people from EWRL and we're very excited about that.
Glen:We've already been chatting with a few of them. There is not yet a concrete plan at the moment, partly because of the other part that you mentioned. Just trying to survive getting past through through some of the stuff where I'll see right now. Because, you know, a lot of us are helping organize the conference. I'm sure some of us are submitting some work to it.
Glen:So it's been a busy last few weeks.
Robin:Do you want to talk about any specific works or themes that you find that you're excited about at RLC?
Glen:There's a particular area that I'm getting more and more excited about these days. And it's actually the complexity these days of where reinforcement learning does really meet deep learning or just some form of function approximation. It's one of the parts I've been considering lately is how complex some of the tasks that the community has been working on historically. So things like the OpenAI Gym have been fantastic for being able to evaluate some algorithms. But in some ways these tasks have still been a bit simple.
Glen:And now that we're branching off into scaling up some of the tasks that we're studying and trying to be able to tackle, we're noticing a lot more challenges to deal with non stationarity, a lot more challenges to deal with continual learning and exploration. So I'm really expecting and hoping for a lot of interesting works that are gonna look at this problem where we're starting to deal a little bit more with scale in terms of task diversity and complexity. We need to revisit how RL and deep learning work together in order to actually make good progress along those directions.
Robin:And I was gonna ask you what you're working on these days. You actually mentioned one topic earlier about, the intersection of supervised learning and and RL, and I see you have a very interesting recent paper on this. So I'm not sure if that's what you're gonna mention, but what what are you working on these days?
Glen:A lot of what I've been working on are types of generalization in planning, which is an interesting area to look into. And yeah, so there's a recent paper I've been working on that has at least been a little bit curious. And the paper is really about trying to understand generalization originally for some of these more recent methods that have been doing supervised learning to try and do planning. So things like decision transformers, and some other methods, we've been calling them.
Robin:A note from Glen. This should read outcome conditioned behavior cloning.
Glen:Outcome-conditioned behavior cloning. So if you base a model where there's some input, that's supposed to condition the actual output of the model. So you can give it a goal and hopefully achieve that. And if we approach this from a supervised learning perspective, is it actually possible to join together some data from one task and another task? So, for the people that are more familiar with doing offline RL and people have been very interested in the problem of being able to stitch trajectories together, which means that if you figure out how to take the go for a walk and find your way to the metro and then take the metro to one location to go home, but somewhat later day you want to take the metro to go to some other place downtown, you can still reuse all the experience from getting from your house to the metro.
Glen:You don't need just that individual trajectory from one specific point and have to have just that whole trajectory. I think humans always do kind of surprisingly well with these types of things and being able to piece together our experiences in order to be able to find a final plan that works well. And at least what we found in the paper is that there isn't currently a mechanism inside of supervised learning methods that are the sequence prediction methods that can do this type of stitching and generalization. Only things that have some type of dynamic programming, things like Q Learning and SARSA have some properties that can enable these. So I've been and that was an interesting finding and part of one of the pieces for generalization I've been looking at.
Glen:I also do a lot of other work in terms of multitask generalization, even some areas for exploration. So, actually and I do have some notions these days that types of good exploration are also really strongly connected to types of very good generalization. So it's these types of problems, that I've been working a lot on recently.
Robin:Do you want to talk about anything else that's going on in RL these days that you find exciting outside of your own work?
Glen:One of the things that's getting me excited is they're really related to some applications for RL. But one specifically is in the area of scientific discovery. And the reason why this area even excites me and I look forward to the problems that come out of this area that are going to be focused on and given to the RL community is there's been a lot of focus lately on supervised learning methods for planning, offline reinforcement learning. Sort of all these mechanisms that make reinforcement learning look more like supervised learning. And as an RL person, this gives me a little bit of concern because it sometimes might cause some people in the community to view exploration as actually a problem with reinforcement learning.
Glen:And I would hope that people can be reminded again that exploration is almost one of the most important features of reinforcement learning and sequential decision making. Only RL can be able to discover new paths and new plans to be able to solve problems. Supervised learning and those other methods, we can train them to be able to reproduce things that we already know how to do really well, which has a lot of merit, but only, like, if we wanna be able to learn things and I'm a scientist. I'm trying to make algorithms that will gather information to be able to give me solutions to stuff that I didn't think was possible. So this is why I think scientific discovery now, which is the making algorithms that can do good exploration to be able to find new designs, novel solutions that we haven't yet found, will be a fantastic area of exploration for reinforcement learning.
Robin:And, is there anything else you wanna share with the audience while while you're here?
Glen:Yes. I mean I'm looking forward to seeing many people at the RL conference this year. I guess like for reinforcement learning, I guess we're all RL people, so I can probably say this and I won't offend too many people. But a lot of the reasons that we wanna do, like, machine learning and decision making is because we wanna eventually have some machines and algorithms that help us make decisions that could have things like that. So there's a lot of other supervised learning problems out there, but the most exciting stuff, even beyond generative models, is to really make decision making agents that will be able to plan and do all sorts of types of AGI and interesting problems into the future.
Robin:I look forward to RLC. You know, the this this show was started. I started the show after, NeurIPS deep reinforcement learning workshop 2018 in Montreal. And, love the vibe and wanted to continue the vibe all year long. And that's why that's that's how the show started.
Robin:So, I look forward to RLC, and I'll make it if I can. And we'll hope to hear more more about this. We'll have a link to your Twitter. And the website, professor Glen Berseth. Thanks so much for doing this today, and we're looking forward to RLC.
Glen:Yeah. No problem, Robert. Thank you.
Creators and Guests
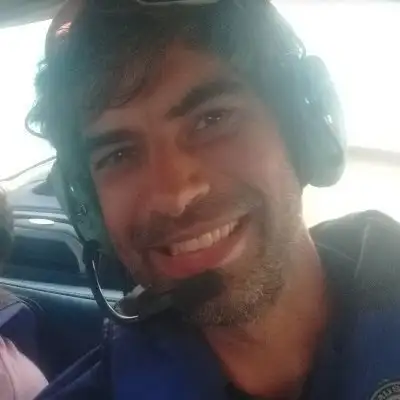
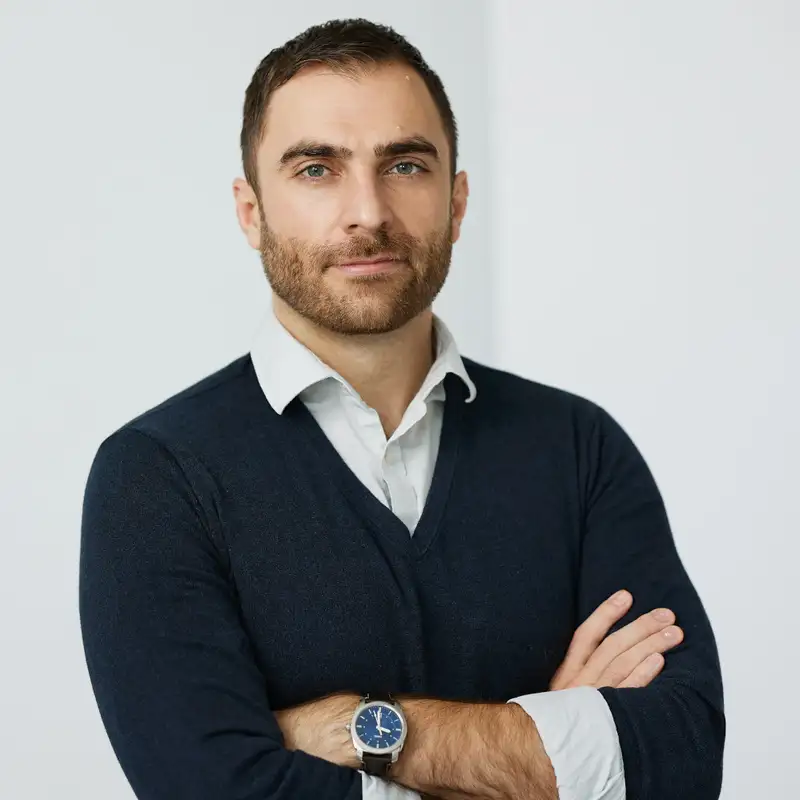