Nan Jiang
Nan Jiang takes us deep into Model-based vs Model-free RL, Sim vs Real, Evaluation & Overfitting, RL Theory vs Practice and much more!
Nan Jiang is an Assistant Professor of Computer Science at University of Illinois. He was a Postdoc Microsoft Research, and did his PhD at University of Michigan under Professor Satinder Singh.
Featured References
- Reinforcement Learning: Theory and Algorithms
Alekh Agarwal Nan Jiang Sham M. Kakade - Model-based RL in Contextual Decision Processes: PAC bounds and Exponential Improvements over Model-free Approaches
Wen Sun, Nan Jiang, Akshay Krishnamurthy, Alekh Agarwal, John Langford - Information-Theoretic Considerations in Batch Reinforcement Learning
Jinglin Chen, Nan Jiang
Additional References
- Towards a Unified Theory of State Abstraction for MDPs, Lihong Li, Thomas J. Walsh, Michael L. Littman
- Doubly Robust Off-policy Value Evaluation for Reinforcement Learning, Nan Jiang, Lihong Li
- Minimax Confidence Interval for Off-Policy Evaluation and Policy Optimization, Nan Jiang, Jiawei Huang
- Empirical Study of Off-Policy Policy Evaluation for Reinforcement Learning, Cameron Voloshin, Hoang M. Le, Nan Jiang, Yisong Yue
Errata
- [Robin] I misspoke when I said in domain randomization we want the agent to "ignore" domain parameters. What I should have said is, we want the agent to perform well within some range of domain parameters, it should be robust with respect to domain parameters.
Creators and Guests
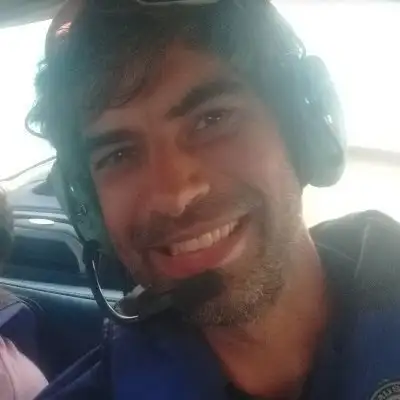
Host
Robin Ranjit Singh Chauhan
🌱 Head of Eng @AgFunder 🧠 AI:Reinforcement Learning/ML/DL/NLP🎙️Host @TalkRLPodcast 💳 ex-@Microsoft ecomm PgmMgr 🤖 @UWaterloo CompEng 🇨🇦 🇮🇳
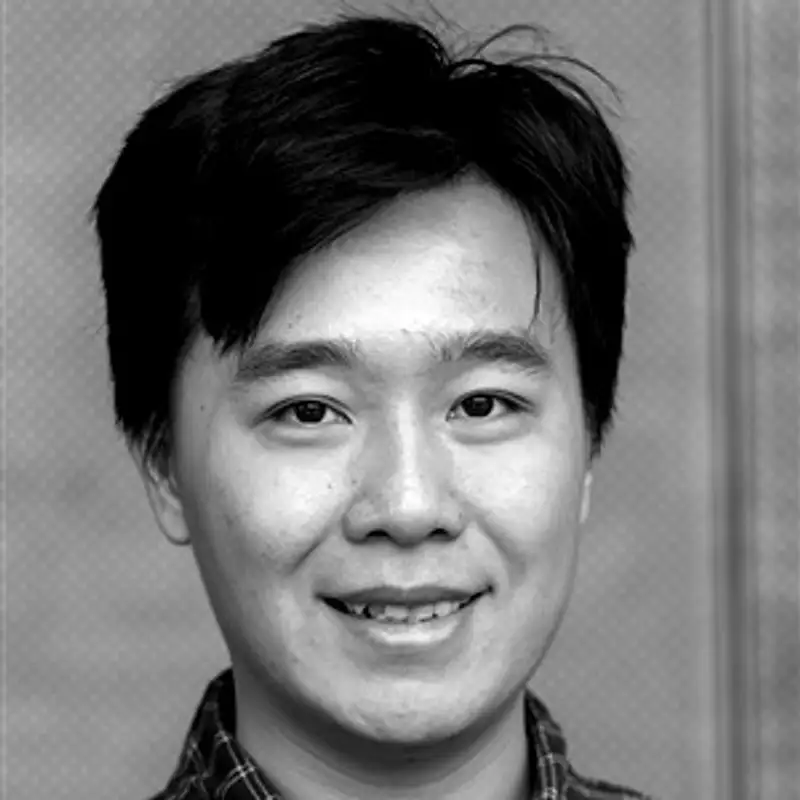